In digital advertising, everyone talks about optimization. As the economy shrinks but our goals don’t, achieving better results by becoming more efficient becomes an increasingly common priority. But, most times, organizations and their agencies fail to really optimize digital ad spending, leaving potential revenue on the table at a time when clients are scarce.
In this article, we will go over key ideas to become more efficient and achieve better results by understanding the key elements of digital advertising campaign optimization.
As an economist that became a digital marketer, when I first faced the issue of digital advertising campaigns optimization, linear programming came into mind. In linear programming, we formulate real-life problems into mathematical models. In short, linear programming is all about achieving linear objectives while facing linear constraints, pretty much what we face in digital advertising.
When doing digital advertising we deal with maximization and minimization problems. For example: maximize the number of students we get from our ad spend given that we have a $100,000 budget. Or, minimize the cost per acquired client from digital campaigns in our e-commerce website subject to the constraint that we need at least 1,000 new clients per month.
Optimization is a mathematical problem about maximizing something (i.e. revenue) and, at the same time, minimizing something (i.e. unitary acquisition costs). And here is where three very common problems arise:
- Not defining correctly what to maximize or minimize (the main goal),
- Not having on-time feedback about how the main goal is performing, and
- Not taking into account all the elements of the mathematical formula involved in achieving our main goal (the restrictions).
We will go over each of these aspects in this article.
What to aim for: correctly defining our optimization goals
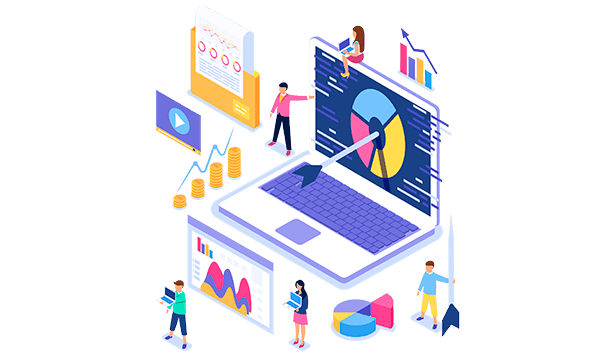
Digital advertising campaigns are normally intended to accomplish important goals such as growth in enrollments, online transactions, gaining first-time purchasers, etc. But, in many cases, when dealing with platforms such as Google Ads or Facebook Ads, we don’t have real-time visualization on those platforms of how the decisions we make impact our main goals.
E-commerce campaigns may be the exception here since at least online revenue is available in real-time (with many caveats). But, for the rest of the industries, this is not possible, due to reasons such as the sales cycle duration or that the goal data might be in other systems, such as a CRM.
Also, in many cases, it takes more than one digital advertising-generated interaction to achieve the sale, so some kind of multi-touch attribution models are required.
Due to these difficulties, most campaigns end up being optimized not to achieve the main goals, but instead other, secondary measures, such as lead form submissions. This is done with the belief that if we maximize leads we will maximize revenue, but this normally doesn’t happen. This is because not all leads are equal. There are truly better leads than others and better lead sources than others.
For example, in higher education, it is common to establish lead maximization as our goal. While this is an important metric and it is fundamental to have enough leads, it also causes us to make mistakes. The goal, instead, should be to maximize the number of enrollments, given a budget. If properly executed, changing the goal will lead to better decisions - and in turn better performance - at each step.
We need to be clear about what we are aiming for and what our top priority is, and put the processes and systems in place to be able to make the right decisions.
Data centralization and measuring what matters
Once we are clear on what we really want to achieve and validate that it is measurable (if not we have problems that go far beyond digital marketing), it is time to start measuring all the relevant data, centralizing it, and generating high-quality indicators.
The most common goal, which is making a sale (a new enrollment, a new client, a new purchase), is frequently first registered in a CRM system of some kind. We need to take the information from each sale we are making and connect it with the effort that generated the corresponding lead, with as much precision and granularity as possible.
Depending on how much we are spending on digital advertising, we should have tens or hundreds of campaigns (distributed on several platforms such as Google Ads), and each should be individually tracked for real goal achievement performance. We need to understand, with as much precision as possible, exactly what is working -- what is generating results.
Digital advertising performance visualization: looking at the big picture
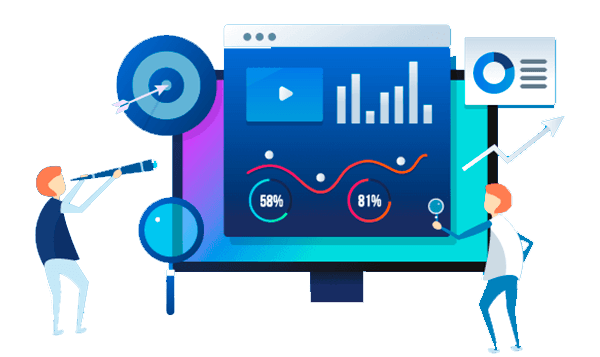
Once we have collected all the real goal data, centralized it and connected it to the individual campaign that generated each sale, it is time to organize this information in a way that enables us to look at it, easily make sense of it, and quickly make the correct decisions.
If we don’t have that much data, as normally happens on campaigns that spend less than two or three million USD per year, we should easily be able to centralize information on online tools such as Google Spreadsheets. But, if the data is large or complex enough, we might need more advanced databases such as Google’s BigQuery. Then, to visualize the data, we could use tools such as Google Data Study, Microsoft Power BI and Salesforce’s Tableau.
But, even if we get the data collection and the technology right, we still might fail to visualize the information correctly. How we construct mixed-sourced KPIs, and how we graph it, or order it, will make all the difference.
We need to be able to see the information and easily know what is working, what isn’t, and what needs more time. We should be able to quickly decide where we are going to reduce budgets, where we are going to increase resources, and where we are going to wait until we have more information. We should also be capable of identifying issues on optimization, configuration, segmentation, etc., so that we can challenge our digital marketers (the guys that actually operate the advertising campaigns).
Optimization is all about math
We need to mathematically understand how the different metrics and actions work in conjunction to help us achieve our goals. Sometimes increasing one number may have a negative impact on another one, therefore resulting in a decrease in overall performance.
For example, it is easy to decrease the cost per lead by investing in some low-cost audiences on Facebook, which may result in a high proportion of unqualified leads, and therefore in a decrease in the lead-to-student conversion rate and an increase in the marketing acquisition cost per student.
For example, I will share a simplified formula for student enrollments through digital ad campaigns:
(Budget) x (CPI) x (CTR) x (site evaporation factor) x (session to lead conversion rate) x (closing rate) = (# of new students).
So, we may do something to increase the CTR of our ads and therefore reduce the cost of our website visitors (equivalently increasing the number of website visitors), but at the same time reduce the session-to-lead conversion rate (getting fewer leads).
The effect of problems with the sales team (or e-commerce site) on optimization
Optimization decisions are made based on data. If the data is corrupted, bad decisions will be made and we won't be able to optimize campaigns.
Even when all the connections and systems work perfectly, there is another source of data corruption (at least kind of) that could lead to wrong decisions.This is the sales team's inefficiencies.
If the sales team is inefficient, and therefore has sub-optimal performance, the data will be distorted by this inefficiency. For example, if the sales teams take too long to contact new leads, or sales people don’t apply appropriate sales techniques or don’t follow up correctly, then we will have low closing rates (percentage of assigned leads that become clients). When optimizing media, this will show up as a subpar performance of the campaign, not of the sales person.
Having an efficient sales team and process is therefore critical to both sales and digital marketing.
Once we understand and mathematically approach digital advertising optimization, with the help of a team of “numbers people” who are also experienced on platforms such as Google Ads and Facebook, achieving better results should be a matter of time.
How can I help?
Let me know if I can be a resource for you in the interests we share (digital marketing and sales/enrollment strategy and execution).
Make sure to book a one-on-one meeting with us by clicking here.
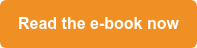